Server Management Using AI Predictions
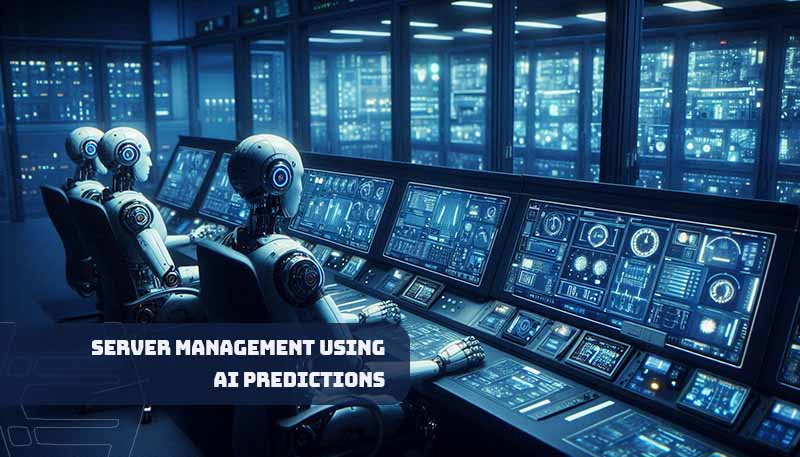

Sharma bal
Table of content
- Introduction
- 1. How AI Predicts Server Issues
- 2. Real-World Applications of AI in Server Management
- 3. Benefits of Server Management via ai predictions
- 4. Challenges and Considerations for Server Management with ai predictions
- Conclusion
Introduction
Server management has evolved significantly over the years, transitioning from manual, labor-intensive tasks to automated, intelligent solutions. While traditional methods have served their purpose, they often fall short in proactively addressing potential issues and optimizing performance. AI predictions are revolutionizing server management by enabling proactive insights, automated actions, and enhanced decision-making.
AI can predict possible server failures, security threats, and performance bottlenecks through the use of machine learning and data analysis. This predictive capability empowers organizations to take proactive measures, such as scheduling maintenance, deploying security patches, or scaling resources, before critical issues arise. With AI predictions, server management shifts from a reactive to a proactive approach, ensuring maximum uptime, optimal performance, and enhanced security.
1. How AI Predicts Server Issues
1.1 Leveraging Machine Learning
The machine learning algorithms present in AI-powered server management, let it analyze historical data. By training these models on past server performance metrics, error logs, and security incident reports, AI pinpoints patterns and anomalies that can lead to potential issues. For instance, AI can recognize correlations between specific system events, such as increased network traffic or resource utilization, and subsequent server failures or security breaches.
1.2 Data Analysis and Insights
To make accurate predictions, AI systems rely on the analysis of various data sources:
- Server Logs: These logs contain detailed information about system events, errors, and warnings. AI algorithms can analyze these logs to identify recurring patterns and potential issues.
- Performance Metrics: Key performance indicators (KPIs) like CPU & memory usage, and disk I/O can be monitored and analyzed to predict potential bottlenecks or performance degradation.
- Network Traffic: Analyzing traffic patterns can be helpful in identifying abnormities, such as unusual spikes or unusual traffic flows, which may indicate security threats or performance issues.
By extracting valuable insights from this data, AI can generate accurate predictions and provide actionable recommendations to prevent problems before they occur.
2. Real-World Applications of AI in Server Management
2.1 Predictive Maintenance
The machine learning predictive capabilities present in AI-powered server management, let it analyze historical data on server performance, hardware components, and environmental factors. It can look for abnormalities, by examining metrics such as:
- Hardware sensor data: Temperature, fan speed, voltage, and power consumption
- Performance metrics: CPU, memory, disk I/O, and network traffic
- System logs: Error messages, warnings, and event logs
For example, if a specific hardware component consistently exhibits abnormal behavior before failing, the AI system can flag it for proactive maintenance.
2.2 Anomaly Detection
AI-powered anomaly detection systems continuously monitor server behavior, identifying deviations from normal patterns. These anomalies can manifest as:
- Sudden spikes in resource utilization: Unusually high CPU or memory usage could indicate a malicious attack or a poorly performing application.
- Unusual network traffic patterns: Abnormal network traffic can be a sign of a security breach or a DDoS attack.
- Frequent system errors or crashes: Recurring system failures can indicate underlying hardware or software issues.
2.3 Capacity Planning
AI capability in analyzing historical data and predicting future workload demands, enable organizations optimize resource allocation. By examining factors such as:
- Historical usage patterns: Analyzing past trends in resource utilization can help predict future needs.
- Seasonal variations: Identifying seasonal fluctuations in workload can help plan for capacity adjustments.
- Business growth: Predicting future growth can inform decisions about scaling infrastructure.
AI algorithms can generate accurate forecasts, enabling organizations to proactively scale their infrastructure to meet future demands. This helps avoid capacity shortages or overprovisioning, leading to cost savings and improved performance.
3. Benefits of Server Management via ai predictions
a. Increased Uptime
- Predictive Maintenance: Identifying and addressing potential failures before they occur can significantly increase uptime. By proactively scheduling maintenance and replacing faulty components, organizations can minimize downtime and ensure uninterrupted service.
- Faster Incident Response: AI-powered monitoring tools can detect issues in real-time, which can accompany by faster response times and lowering downtime.
b. Improved Performance
- Optimized Resource Allocation: AI can analyze historical data and real-time usage patterns to optimize resource allocation. Dynamic adjustment of resource allocation based on workload demands, let AI improve overall system performance and responsiveness.
- Automated Performance Tuning: AI can automatically fine-tune system parameters, such as CPU frequency, memory allocation, and network settings, to optimize performance.
c. Cost Savings
- Reduced Operational Costs: AI-driven automation can lead to significant cost savings by reducing the need for manual intervention.
- Optimized Resource Utilization: By accurately predicting resource needs, AI can help organizations avoid overprovisioning, leading to lower infrastructure costs.
- Improved Energy Efficiency: Identifying and addressing inefficiencies via AI-powered systems can lead to energy consumption optimization.
d. Enhanced Security
- Proactive Threat Detection: AI-based security measures can help in real-time diagnosing and responding to threats, preventing cyberattacks and data breaches.
- Automated Security Patching: AI can automatically identify and deploy security patches, reducing the risk of vulnerabilities.
- Anomaly Detection: What AI sees as abnormal patterns in network traffic and system behavior, can be an indication of potential security threats.
Note: Despite the differences among specific figures and statistics in organizations and the specific AI implementation, studies have shown that AI-powered server management can accompany with meaningful improvements in uptime, performance, and security. For instance, some organizations have reported a reduction in downtime by up to 50% and a 20% increase in server performance after implementing AI-powered solutions.
4. Challenges and Considerations for Server Management with ai predictions
While AI offers significant benefits for server management, several challenges and considerations must be addressed:
4.1 Data Quality and Quantity
- Data Bias: AI predictions are heavily impacted by the training data, and any bias in them can make predictions inaccurate. Having representative and unbiased data is essential for training models.
- Data Noise and Inconsistency: Noisy or inconsistent data can negatively impact the accuracy of AI predictions. Data cleaning and preprocessing techniques are essential to ensure data quality.
- Data Privacy and Security: To protect against unauthorized access and data breaches, it is necessary to implement robust security measures when handling sensitive server data.
4.2 Model Complexity
- Overfitting complex AI models to the training data can result in poor performance on new, unseen data. Regularization techniques can help mitigate this issue.
- Interpretability: While complex models can make accurate predictions, they may be difficult to interpret. This can hinder understanding the underlying reasons for predictions and limit the ability to debug and improve the model.
4.3 Integration with Existing Infrastructure
- Legacy Systems: Integrating AI-powered solutions with legacy infrastructure can be challenging, requiring careful planning and customization.
- Data Compatibility: Ensuring compatibility between AI systems and existing data sources can be complex, especially when dealing with diverse data formats and standards.
- Organizational Change Management: Implementing AI-powered server management requires a cultural shift and may necessitate training and education for IT staff.
Conclusion
As AI technologies continue to advance, we can expect even more sophisticated applications in this domain. From predictive analytics and automated remediation to autonomous systems, AI will revolutionize the way we manage and maintain server infrastructure.
Organizations seeking to optimize their IT operations must embrace AI because it’s not just a trend; it’s a strategic imperative. Businesses can achieve greater efficiency, reliability, and security by utilizing the power of AI.
Hostomize is committed to leveraging AI to deliver cutting-edge hosting solutions. Our AI-powered platforms are designed to provide optimal performance, security, and scalability.
To learn more about how AI can transform your server management, explore our website or contact our experts.